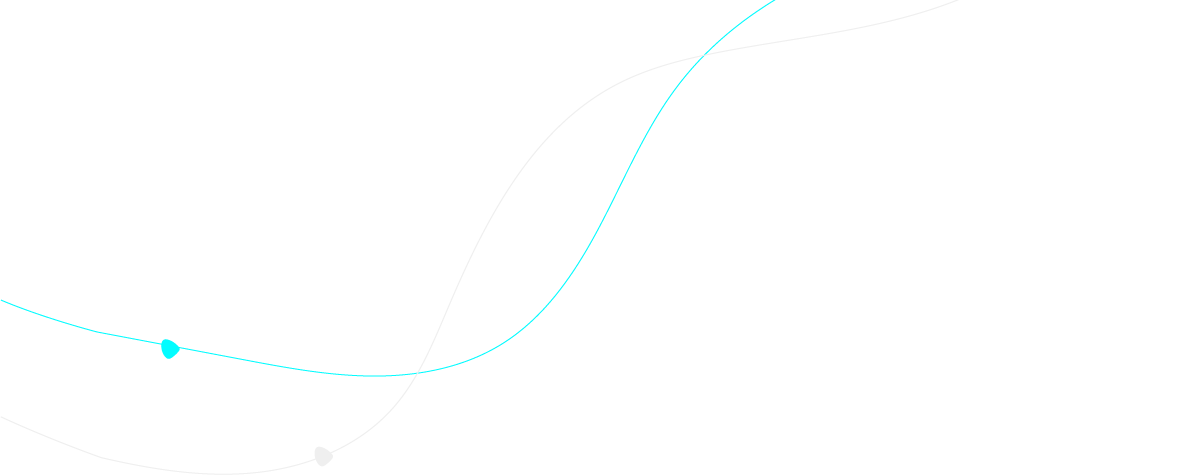
Time to improve the quality of your data annotation process?
White PaperMastering Annotation for Complex Data: Insider Tips and Tricks for CV and NLP
Expert Insights: Lessons Learned from Over 100 AI Projects
There are several main issues that can arise when annotating complex data:
- Lack of standardization: Different annotators may interpret the annotation task differently, leading to inconsistent or inaccurate annotations.
- Scalability: Annotating large amounts of data can be time-consuming and resource-intensive.
- Inter-annotator agreement: Different annotators may disagree on the annotation of certain instances, leading to a lack of consistency in the data.
- Quality control: Ensuring the accuracy and consistency of annotations can be difficult, particularly when working with large amounts of data.
- Human error: Annotators may make mistakes or introduce biases, leading to inaccuracies in the data.
- Data privacy and security: Annotating sensitive data requires strict controls to protect the privacy and security of the information.
- Lack of domain expertise: Annotators may not have the necessary domain knowledge to accurately annotate certain types of data.
We have not been able to confirm your registration.
Your registration is confirmed.
By clicking submit you agree to our Privacy Policy.
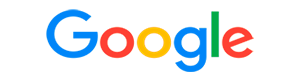
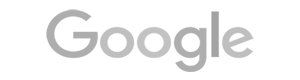
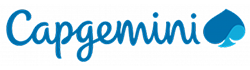
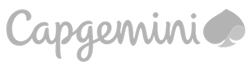
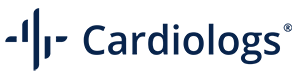
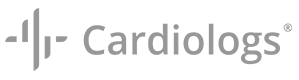
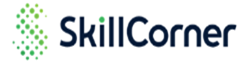
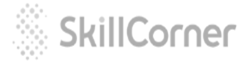
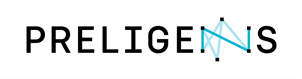
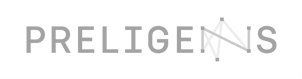
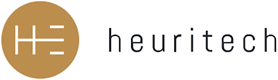
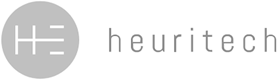
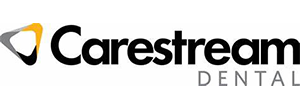
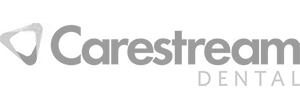
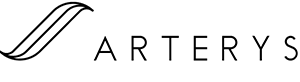
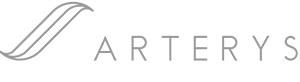
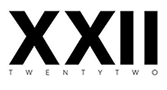
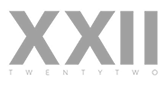
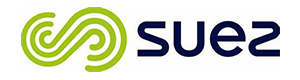
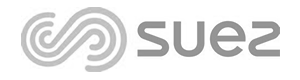
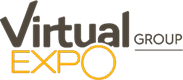
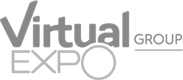
Why an AI business in Healthcare needs ?
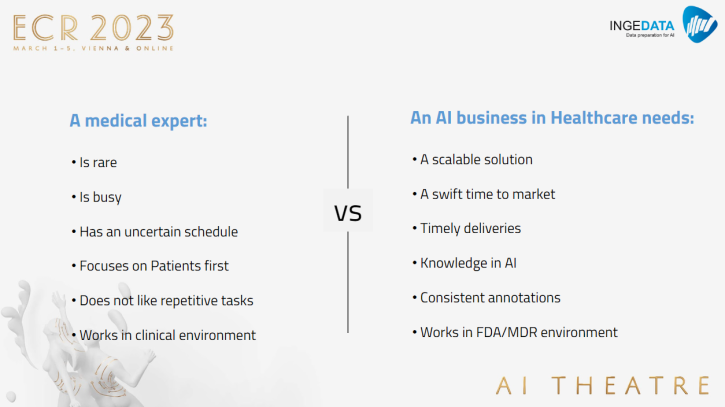
"Annotation is the most time-consuming and expensive part of creating a machine learning model, but it is also the most important.”
Andrew Ng, Co-founder of Google Brain, Co-founder of Coursera